- TOP
- Cutting-edge Research and Social Contribution
- Cutting-edge Research
- Updating agriculture with ICT technology: A new, more advanced type of image recognition technology based on deep learning
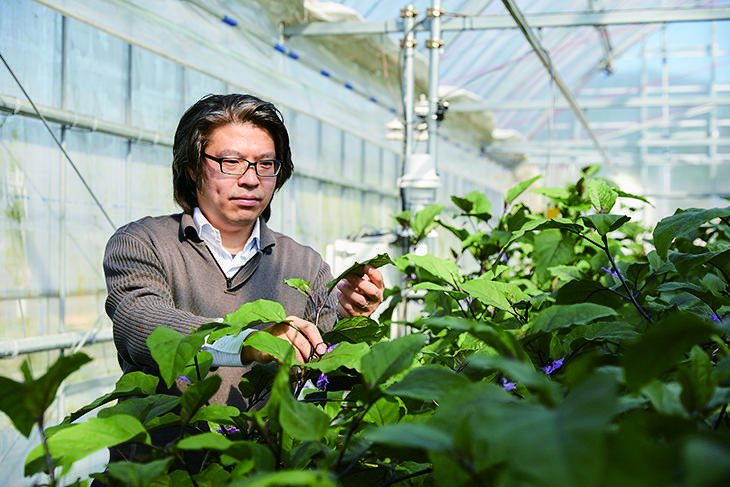
Updating agriculture with ICT technology: A new, more advanced type of image recognition technology based on deep learning
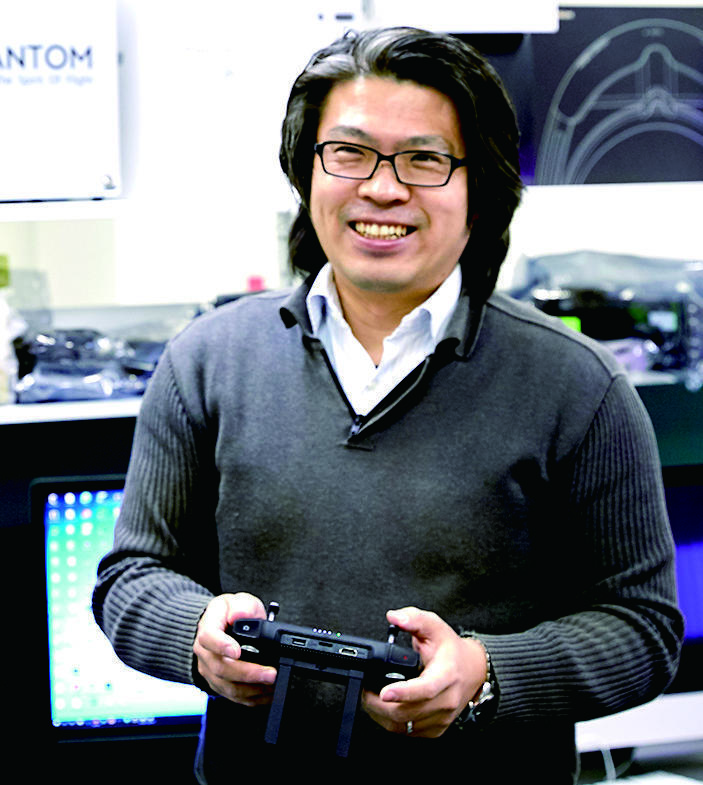
KURIHARA Toru
- Specialized field
Image measurement, optical measurement, measurement engineering
Correlation image sensors allow temporal correlation between incident light and reference signals, a type of signal processing technology, inside of a camera. Associate Professor Toru Kurihara has achieved results by researching three-dimensional measurement and shape inspection techniques using these special sensors. Since 2017, he has participated in Advanced Next-Generation Greenhouse Horticulture by IoP (Internet of Plants), a Cabinet Office project promoted by our university. To improve detection precision of fruits and vegetables--a key point for automation of agricultural work--Kurihara is developing more advanced image recognition technology by using not only ordinary color images, but also additional information such as spectra and thermography.
Design of optical filters to realize improved detection precision with RGB cameras
As the decline in the Japanese agricultural population worsens and attention turns to automation of agricultural work using machinery, active efforts are being made to develop automatic harvesting robots to replace humans in time-consuming harvesting work. Many of the techniques employed here detect fruits using images taken by ordinary cameras and expressed with the three RGB colors. However, green fruits similar in color to leaves and stems, like bell peppers, are difficult to detect using RGB images, and obstacles to automation remain significant. Hyperspectral cameras have previously been used to identify fruits like this which are similar in color to the background color. With these cameras, it is possible to acquire spectral intensities for each pixel with high wavelength resolution, and recognize even small differences in spectral intensity that cannot be confirmed with an ordinary camera or human vision.
On the other hand, hyperspectral cameras are expensive, and difficult for farmers to widely adopt. Kurihara thought that, to improve identification precision of hard-to-detect bell peppers, "we can extract effective information from hyperspectral data, while using inexpensive RGB cameras, and thereby provide a framework for making maximal use of that information." Thus, he proposed equipping RGB cameras with optical filters that enable extraction of wavelengths effective for identifying bell peppers. He devised new techniques using deep learning technology to design these optical filters.
"Information truncated to the three colors of RGB was the starting point in previous deep learning, but in the real world, there is infinite-dimensional spectral information. My idea was to reconstruct a neural network as a framework for making good use of this information."
As Kurihara says, conventional deep learning is premised on input of an RGB color image, and researchers tried to improve identification capability by devising network structures after that stage. With this new technique, Kurihara decided to jettison that premise, take a hyperspectral image as input, place an optical filter prior to the RGB color filter, and adopt a structure enabling selection of wavelengths useful for identification. Another key point was designing the optical filter through learning, by inserting RGB color filters into the deep learning network with fixed weights. Using this approach, it was possible to achieve simultaneous optimization through deep learning of all of the following: selection of wavelengths by optical filters realized as learnable weights, and a segmentation network taking as input a color image wherein each pixel is truncated to three colors.
"Ordinary cameras have varying wavelength sensitivity depending on the model, and thus RGB color filters must be fixed in order to simultaneously design an optical filter and neural network effective for a specific camera. That is, if in the deep learning process, we define RGB color filters as fixed weights whose values do not change due to learning, then learning proceeds simultaneously for both learnable, variable optical filter weights, and the neural network for recognition whose starting point is RGB, and it is possible to simultaneously design an optical filter and recognition part optimal for the camera model to be used."
By using the proposed method, hyperspectral images of growing bell peppers were captured, learning data was created for each pixel from those images, and identification was carried out. As a result, an improvement of about 2% in precision was achieved. That is, Kurihara successfully derived optical filter coefficients enabling an improvement of 2% in precision. His aim is to collaborate with companies to produce optical filters designed based on these results, and achieve development as a new architecture for smartphones.
Development of fruit and vegetable detection methods employing thermography cameras and temperature differences
Identifying fruits by their color and shape is required not only for automation of harvesting, but also for operations such as harvest prediction and understanding of the growth situation. In order to detect fruit that is hard to detect with ordinary cameras, Kurihara is also researching techniques using time series variation of thermal images captured with thermography cameras. Generally speaking, it is thought that among objects made of the same material, those with larger volume vary less in temperature, and fruits tend to vary less in temperature than leafs and stems. Thus Kurihara carried out long-term photography of growing eggplants using a thermography camera, and as a result, found that temperature of fruits changes slowly compared to leaves and stems. He succeeded in separating fruit from leaves and stems by fitting time series variation in temperature over two hours at sunset to an exponential function.
There are also cases where a rise in the temperature of plant leaves is evident due to disease-caused crop damage or environmental stress, and thus a thermography camera was applied to early detection of ginger rhizome rot, a disease affecting ginger which causes a great deal of harm. Plants with and without the disease were photographed continuously over a fixed period of time, and when the measurement data was analyzed, a trend was evident where leaf temperature was higher for plants with the disease, starting from 2-4 days before onset was confirmed, and this showed it is possible to confirm disease onset far more quickly than through visual inspection by people.
Kurihara: "By placing a color camera and thermography camera at the same location and creating data in which a color image is overlaid with a thermography image, as studied in this research, we can configure a neural network with four channels (RGB and thermal), and there is high potential for further improvement in detection precision." Apparently, higher-precision recognition technology can be developed by combining spectral and thermography elements with color images.
"My goal for the future is to develop inexpensive, state-of-the-art automatic harvesting robots together with people from companies interested in high-precision recognition technology."